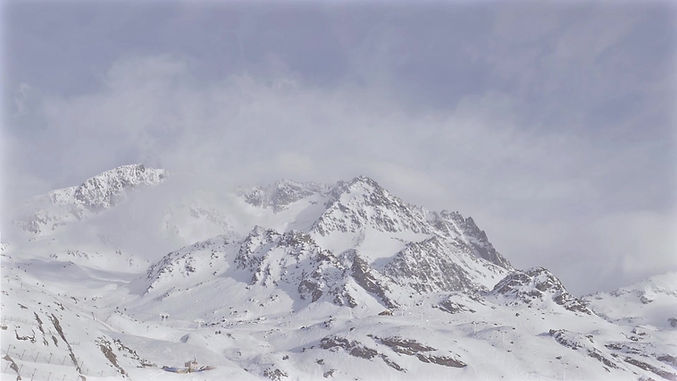
Our Vision
Our academic group is dedicated to advancing the fields of AI, machine learning, and NLP while focusing on real-world business problems. Our vision is to solve new and challenging business problems using cutting-edge research and end-to-end development of innovative methods and solutions that drive business outcomes. Our solutions have a business impact across diverse industries such as Healthcare, HR, Fintech, Social Media, Human-AI Interaction, Crowdsourcing, and Law.
Lab Chairs
Prof. Inbal Yahav
Prof. Inbal Yahav is an accomplished expert in developing ML and NLP architectures with a background in CS and data mining. Her work is driven by a passion for interdisciplinary research, leading to exciting collaborations with the Department of Law and Middle East Studies. She also serves as an editor for INFORMS Journal on Data Science and is one of the authors of the textbook "Machine Learning for Business Analytics: Concepts, Techniques, and Applications in R". As a trusted Data Science consultant, she works with leading organizations such as the Bank of Israel, Midgam Research & Consulting Ltd., and the Ministry of Education.
Dr. Tomer Geva
Tomer Geva is an experienced machine learning and data science researcher with a focus on solving business problems and developing methods to improve predictive accuracy. He is a tenured senior lecturer at Tel-Aviv University and founder of its Business Data Science Program, which he led for six years. Tomer's research has been published in leading Machine Learning and Management journals, and he has received generous funding from various foundations and companies, including the Israel Science Foundation and Google. Tomer serves as an editor for leading Information Systems journals, including MIS Quarterly and Decision Support Systems. He has extensive industry experience, having worked with high-tech companies, financial organizations, and the public sector in the fields of Machine Learning, AI, NLP, and Data Science.
Dr. Moshe Unger
Dr. Moshe Unger is an expert in developing machine learning, deep learning and AI methods to solve business problems. His research focus in recommender systems and developing data science methods that safeguard privacy while enabling organizations to make informed decisions based on big data. He earned his doctorate from Ben Gurion University and completed a post-doctorate at New York University's business school. He has managed multiple projects at the Cyber Security Research Center and worked collaboratively with leading companies, including Spotify and Dell EMC. Additionally, Dr. Unger currently serves as an Amazon visiting academic.
Meet Our Students
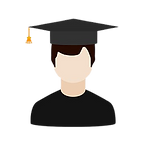
Avihay Chriqui
PhD Student
Avihay Chriqui
Advisor: Prof. Inbal Yahav
Dissertation title: NLP Tools for User Generated Content Monitoring
Published papers: link
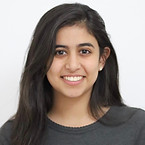
Rawan Qadri
PhD Student
Rawan Qadri
Advisors: Prof. Inbal Yahav and Dr. Moshe Unger
Thesis title: Towards Aspectual Entailment-Informed Recommender Systems
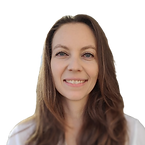
Yulia Nudelman
PhD Student
Yulia Nudelman
Advisors: Prof. Inbal Yahav and Prof. Dan Amiram
Dissertation title: TBD
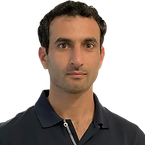
Nir Cohen
Masters Student
Nir Cohen
Advisor: Dr. Moshe Unger
Thesis title: Situalization in Recommender Systems
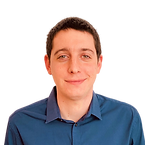
Or Zuckerman
PhD Student
Or Zuckerman
Advisor: Dr. Moshe Unger
Dissertation title: Privacy-Aware Context-Aware Recommender Systems

Tom Sharib
Masters Student
Tom Sharib
Advisor: Dr. Moshe Unger
Thesis title: Cross Domain Customer Representation

Chen Elyashar Shedletzky
PhD Student
Chen Elyashar Shedletzky
Advisors: Prof. Inbal Yahav and Dr. Sagit Bar-Gil
Dissertation title: Managing Chatbot Interactions Through Sentiment Analysis

Tim Shektov
Research Assistant
Tim Shektov
Advisor: Prof. Inbal Yahav
Thesis title: Named-Entity Recognition for the Hebrew Legal Domain
Former students
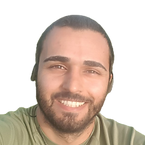
Shahar Meir
Masters Student
Shahar Meir
Advisors: Dr. Tomer Geva
Thesis title: Automatic Short Answers Grading for Workers Evaluation
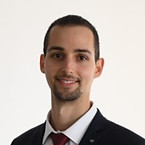
Amit Kupitz
Masters Student
Amit Kupitz
Advisor: Dr. Inbal Yahav
Thesis title: Rannox: A Reciprocal Human-Machine Interaction Framework for The Annotation and Examination of Textual Data
Tools and Datasets
Welcome to our "Tools and Datasets" section, where you'll find an updating list of open-source tools and datasets available for free under the GNU license. Our collection of resources is designed to support researchers, developers, and data enthusiasts in pursuing innovation and discovery. Feel free to browse, download, and contribute to these valuable assets.
A Pre-trained BERT for Polarity Analysis and Emotion Recognition.
Dataset: we collected Israeli news site comments, and labeled sentiment and emotion conveyed in the comments. The data is available in this link.
A Hebrew version of our paper is now available here. The paper also features a tutorial on sentiment analysis and emotion detection in Hebrew.
A preliminary BERT model for Hebrew legal and legislative domains.
Dataset: we made part of our dataset available for public use in this link.
We are currently in the process of collecting and annotating more legal documents! You can find more here. If you wish to join us, please contact us via email.
The recordings of the legal language analysis symposium that took place on June 15th, 2023 have been uploaded to YouTube. Check them out at this link!
Fusion is a software system designed to facilitate Reciprocal Human-Machine Learning (RHML), specifically tailored for text classification tasks. This innovative platform enables a symbiotic relationship between humans and machines, allowing each to learn from the other to improve overall performance and accuracy in classifying texts.
Stay tuned! We are currently working on the second generation on Fusion, and on multiple projects that utilize the RHML framework.
New! See our article in Globes that explains the risks of keeping humans out of the learning loop here.
An innovative project based on our research is currently being submitted to the AI Designers for Democracy competition at the Shamgar Center for Digital Law and Innovation. You can find more information in this link.
Selected Publications
NEW! Reciprocal Human-Machine Learning: A Theory and an Instantiation for the Case of Message Classification
Dov Te'eni, Inbal Yahav, Alexey Zagalsky, David Schwartz, Ghal Silverman, Daniel Cohen, Yossi Mann, and Dafna Lewinsky (2023), Management Science. link
NEW! Predicting consumer choice from raw eye-movement data using the RETINA deep learning architecture
Unger, M., Wedel, M. & Tuzhilin, A. Predicting consumer choice from raw eye-movement data using the RETINA deep learning architecture. Data Min Knowl Disc (2023). https://doi.org/10.1007/s10618-023-00989-7 link
Moshe Unger, Pan Li, Sahana Sen, and Alexander Tuzhilin (2023), ACM Transactions on Management Information Systems. link
HeBERT and HebEMO: A Hebrew BERT Model and a Tool for Polarity Analysis and Emotion Recognition
Avihay Chriqui and Inbal Yahav (2022), Informs Journal on Data Science. link
Legal HeBERT: A BERT-based NLP Model for Hebrew Legal, Judicial and Legislative Texts
Avihay Chriqui, Inbal Yahav, and Ittai Ber-Siman-Tov (2022). link
Who is a Better Decision Maker? Data‐Driven Expert Ranking Under Unobserved Quality
Tomer Geva and Maytal Saar‐Tsechansky (2021), Production and Operations Management. link
The Design of Reciprocal Learning Between Human and Artificial Intelligence
Alexey Zagalsky, Dov Te'eni, Inbal Yahav, David G. Schwartz, Gahl Silverman, Daniel Cohen, Yossi Mann, and Dafna Lewinsky (2021), Proceedings of the ACM on Human-Computer Interaction. link
Tomer Geva and Inbal Yahav (2021), IEEE Transactions on Knowledge and Data Engineering. link
Moshe Unger, Alexander Tuzhilin, and Amit Livne (2020), ACM Transactions on Management Information Systems. link
Moshe Unger and Alexander Tuzhilin (2020), IEEE Transactions on Knowledge and Data Engineering. link
Inbal Yahav, Onn Shehory, and David Schwartz (2019), IEEE Transactions on Knowledge and Data Engineering. link
More for Less: Adaptive Labeling Payments in Online Labor Markets
Tomer Geva, Maytal Saar-Tsechansky, and Harel Lustiger (2019), Data Mining and Knowledge Discovery. link
The insider on the outside: a novel system for the detection of information leakers in social networks
Giuseppe Cascavilla, Mauro Conti, David G Schwartz, Inbal Yahav (2018), European Journal of Information Systems. link
Inbal Yahav, and Galit Shmueli (2017), Production and Operations Management. link
Inbal Yahav, Galit Shmueli, and Deepa Mani (2016), MIS Quarterly. link
Erik Brynjolfsson, Tomer Geva, and Shachar Reichman (2016), MIS Quarterly. link
Moshe Unger, Ariel Bar, Bracha Shapira, and Lior Rokach (2016), Knowledge-Based Systems. link